2022.08.23
Emotion Analysis for Tweets Regarding COVID-19 Vaccines
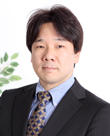
- Hidetsugu Nanba
- Professor, Faculty of Science and Engineering, Chuo University
Area of Specialization: Natural Language Processing
People's anxiety and risk communication
While new technologies enrich our lives, their newness creates uncertainties that existing knowledge cannot handle. Not limited to technology, people are worried about unpredictable situations such as natural disasters and climate change. In order to eliminate such anxiety, risk communication between the parties concerned regarding the factors of the anxiety is indispensable.
For example, in the case of COVID-19, the parties concerned refer to the general public, employees at pharmaceutical companies, government officials, experts, etc. who inoculate the vaccine. Some citizens may be skeptical about the safety of the vaccine, and others may be dissatisfied with how vaccination tickets are distributed. If people are dissatisfied with the distribution of vaccination tickets, it is necessary to consider how to grasp such information, how experts can convey information such as vaccine safety to people in an easy-to-understand manner and how the government will resolve the dissatisfaction.
I am currently involved in a project called sense of security management with researchers in various fields. As safety and security are required in every aspect of society, it is first necessary to objectively grasp the sense of security and anxiety that people have as a prerequisite for management discussions. My specialty is natural language processing, which, to put it simply, is research that enables computers to handle the words that humans normally use. It is a field of artificial intelligence, and in recent years, it has been increasingly taken up by the mass media in the form of big data analysis using AI. My role in this project is to use this natural language processing to find out the various anxieties that people have on the Internet. In the following, I will first explain the method of sentiment analysis using natural language processing, and then the method to analyze what people are feeling relieved or anxious about, and on what grounds, for Twitter posts.
Emotion analysis using natural language processing
In natural language processing, many studies have been conducted to analyze people's emotions, targeted at free answer questionnaires, reviews of online shopping sites, and postings on SNS. In that early study, it was common for computers to create emotional word dictionaries in advance in order to classify texts and sentences, and to estimate emotions based on the presence or absence of words in the dictionary. For example, suppose that words such as "worry," "anxiety," and "sorrow" are registered in the "anxiety" category of the emotional word dictionary, and the following sentence (1) is processed.
(1) I'm not against vaccination, but I haven't even made a reservation because I'm worried that my immunity will drop on the contrary to the third hit.
Since this sentence contains the word "worry," the computer can refer to the emotional dictionary and determine that this is a sentence in the "anxiety" category.
Although such a dictionary-based method is simple, it is effective in understanding the general tendency of the entire text set. However, there are the following problems.
(Problem 1) Even if an emotional word is included in the text, the text and the emotional category do not always match.
(2) The media has long fueled anxiety about vaccines, so I think that's part of it.
Although the word "anxiety" is included in this sentence (2), the author of this sentence does not feel anxious. There is also the opposite pattern.
(Problem 2) Emotional words are not included, but the whole sentence or text can be classified into a specific emotional category.
(3) A mother who was vaccinated without any doubt after being told by her doctor that she was okay. After her first vaccination, she was raced to a hospital twice. She is still having sleepless days while freaking out about a sudden change. I lost my younger brother in a medical accident, and I now can't believe the words that "the odds are low" or "it's rare."
This sentence (3) does not include emotional words ("worry," "anxiety," or "sorrow") classified in the anxiety category of the emotional word dictionary. However, if you read the whole sentence, you can see that this author feels uneasy.
Regarding the above problems, it is necessary to have a mechanism in which a machine captures the meaning of the sentence, rather than focusing only on the individual words in the sentence. In this respect, by using the language model using transformer[1] including BERT[2], a considerable part is being resolved. This is because it has become possible to grasp the structure of a sentence from various aspects to some extent by using a mechanism called the self-attention mechanism in a transformer. In fact, the above sentence (3) can be judged to be a sentence in the "anxiety" category even on a computer by using the sentiment analysis model[3] using BERT constructed by the authors.
Analysis of changes in people's emotions toward vaccines during COVID-19 and the factors behind those changes
Analysis of changes in people's emotions toward vaccines during COVID-19 and the factors behind those changes
Let me introduce an example of collecting tweets about the new coronavirus vaccine from Twitter and analyzing tweets that have anxiety about the vaccine. For details of the analysis, please refer to Reference[3].
The authors collected tweets posted on Twitter between June 13, 2021 and November 30, 2021. We analyzed Japanese tweets in Japan and English tweets in other countries, targeting six countries: Japan, the United States, the United Kingdom, Canada, Australia, and India. Table 1 shows the number of tweets in each country. The analysis examines how the emotion ratio changes over time after automatically classifying each tweet into eight emotional categories (joy, sadness, expectation, surprise, anger, fear, disgust, and trust) (Figure 1 to Figure 6). Regarding the timing of sudden changes, we investigated why the changes occurred by using the dependency analysis and burst detection algorithm[4] (Table 2). In this analysis, "fear" and "disgust" correspond to anxiety.
Figure 1 shows the transition of emotions in Japan. Looking at this figure, the three emotions of "joy," "sadness," and "fear" fluctuate significantly during the period from June 13 to July 31. Therefore, these tweets were scrutinized by using the dependency analysis, and word pairs having a dependency relationship that increased rapidly during this period were extracted by using burst detection. The results are shown in Table 2. From June 13 to July 31, vaccination began fully, and it can be seen that there are many tweets about "joy" regarding the arrival of vaccination tickets or the reservation of vaccination. On the other hand, during this period, pitcher Yusuke Kinoshita of the Chunichi Dragons died after vaccination, and although the causal relationship with the vaccine is unknown, it can be seen that many people felt sadness or fear.
Comparing emotional trends by country, Japan has the highest rate of tweets about fear (Figure 1), while the other four countries other than India have a high rate of anger and disgust. The reason why only Japan had a different tendency is not clear from the results of analyzing tweets. In the future, it is necessary to carry out further analysis in combination with the knowledge obtained in fields such as psychology.
Table 1 Number of tweets by country
Name of country | Number of tweets |
Japan |
24,292,412 |
United States |
325,743 |
United Kingdom |
58,462 |
Canada |
47,097 |
Australia |
35,274 |
India |
32,337 |
Figure 1 Ratio of tweets to which emotion labels were assigned (Japan)
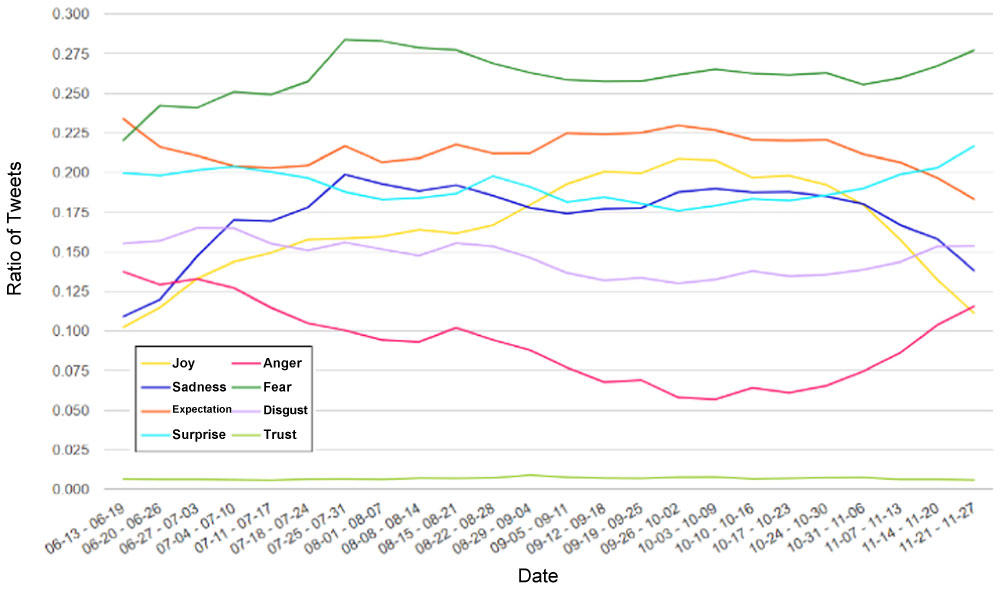
Figure 2 Ratio of tweets to which emotion labels were assigned (United States)
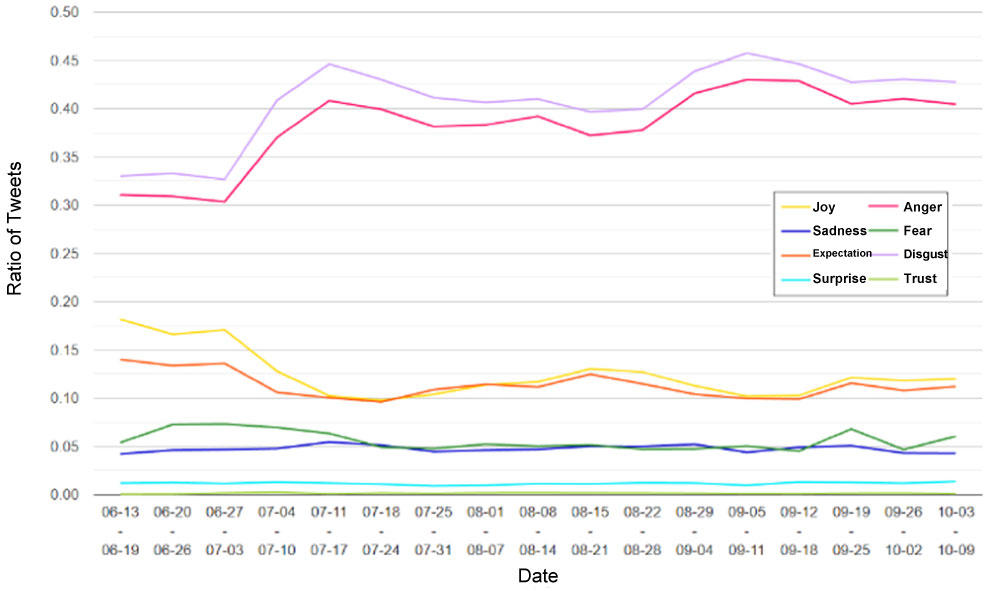
Figure 3 Ratio of tweets to which emotion labels were assigned (United Kingdom)
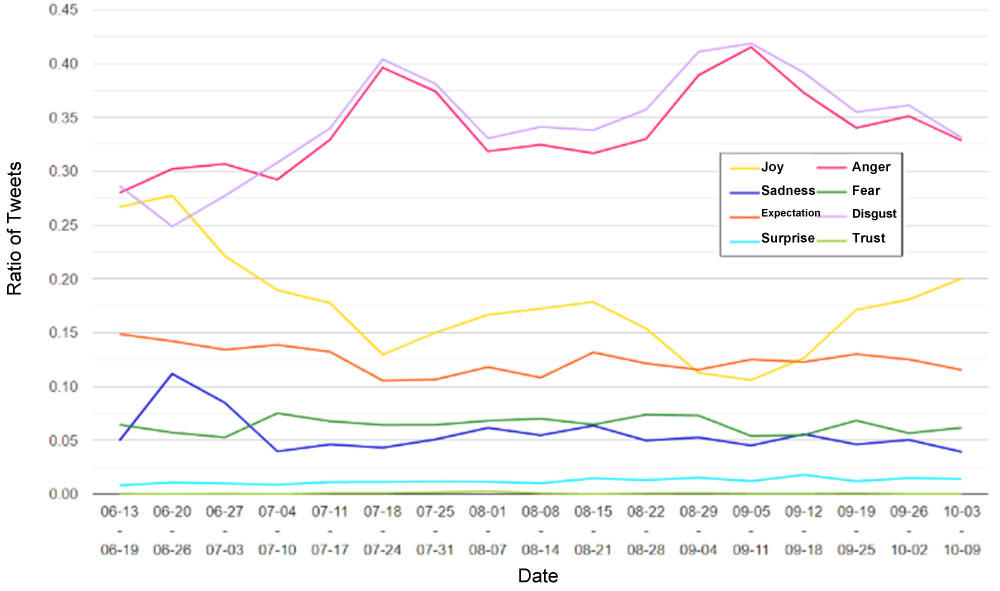
Figure 4 Ratio of tweets to which emotion labels were assigned (Canada)
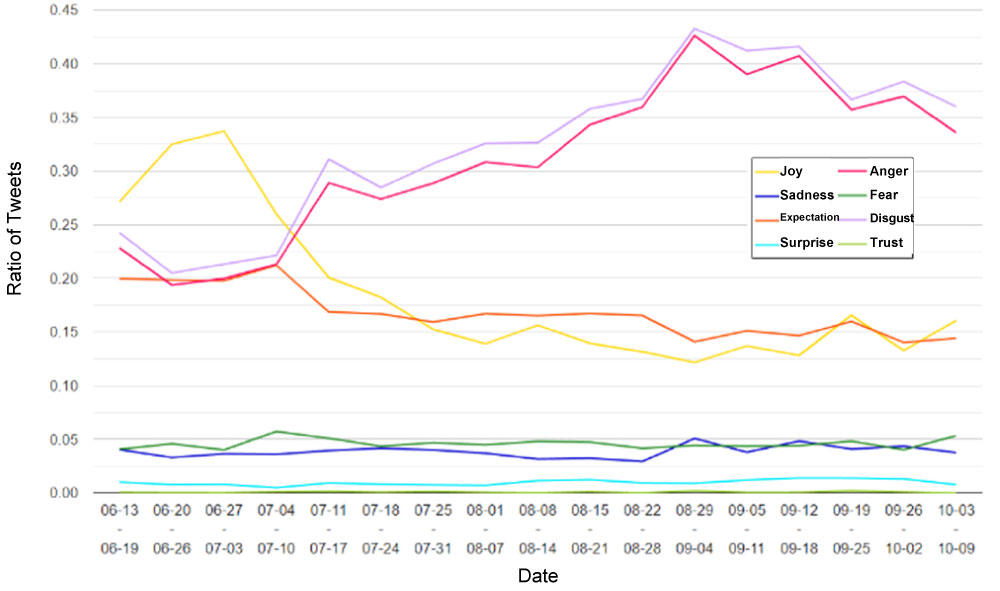
Figure 5 Ratio of tweets to which emotion labels were assigned (Australia)
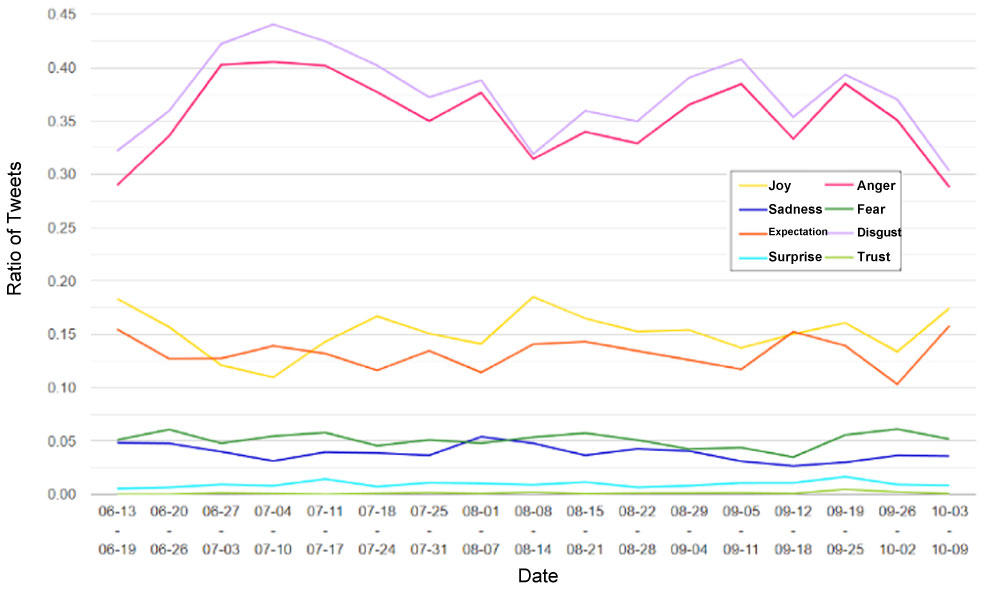
Figure 6 Ratio of tweets to which emotion labels were assigned (India)
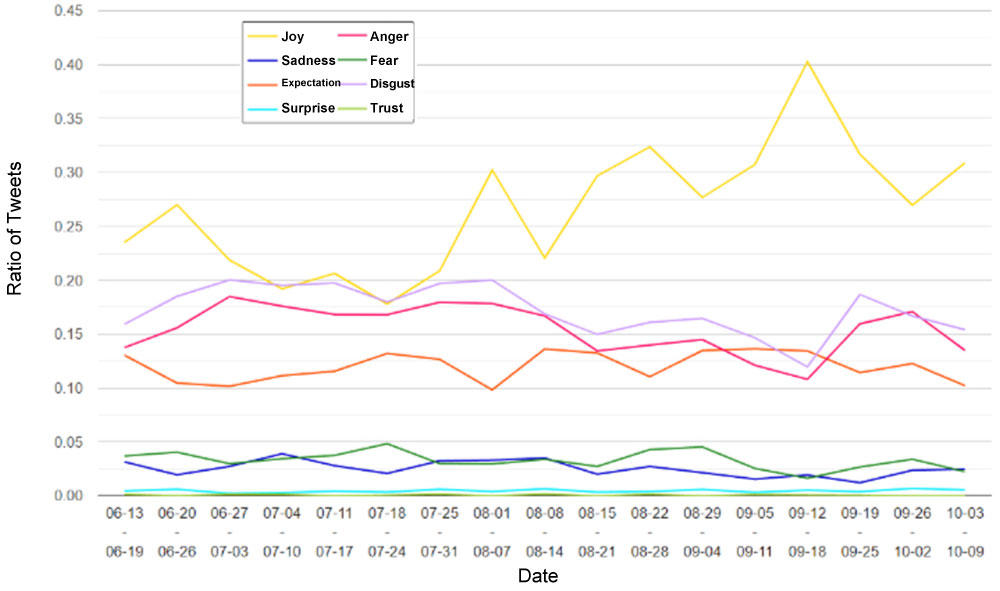
Table 2 Dependency relationship in Japan as determined by burst detection
(June 13, 2021 to July 31, 2021)
Emotion | Dependency relationship | Number of tweets |
Joy |
(Vaccination, appointment) |
7278 |
(Vaccination ticket, receipt) | 6448 | |
(Vaccine, ticket) | 5277 | |
(vaccination ticket, receipt) | 4604 | |
(Vaccination, done) | 988 | |
(2nd dose, done) | 438 | |
Sadness | (Vaccine, shot) | 15045 |
(Yahoo, news) | 7654 | |
(Vaccination ticket, receipt) | 6187 | |
(Vaccine, ticket) | 5193 | |
(Vaccination ticket, receipt) | 5106 | |
(Vaccine, insufficient) | 3350 | |
(COVID-19, infection) | 2734 | |
(Kinoshita, Yusuke) | 2333 | |
Fear | (Yahoo, news) | 20262 |
(Vaccination, no progress) | 11412 | |
(COVID-19, infection) | 9701 | |
(Vaccine, effect) | 9575 | |
(COVID-19 vaccine, vaccination) | 7218 | |
(Infected person, increase) |
6889 | |
(Kinoshita, Yusuke) | 4192 | |
(COVID-19, scary) | 3709 |
Reference
[1] Devlin, J., Chang, M.-W., Lee, K., and Toutanova, K.: BERT: Pre-training of Deep Bidirectional Transformers for Language Understanding, Proceedings of the 2019 Conference of the North American Chapter of the Association for Computational Linguistics: Human Language Technologies, Vol. 1, 4171-4186, 2019.
[2] Vaswani, A., Shazeer, N., Parmar, N., Uszkoreit, J., Jones, L., Gomez, A.N., Kaiser, L., and Polosukhin, I.: Attention Is All You Need, Proceedings of the 31st International Conference on Neural Information Processing Systems, 6000-6010, 2017.
[3] Satoshi Fukuda, Hidetsugu Namba, Yuko Shoji, Analysis of People's Emotional Changes and Their Factors for Vaccines in the Novel Coronavirus Pandemic, Journal of Japan Society for Fuzzy Theory and Intelligent Informatics, 2022. (Scheduled to be posted)
[4] Kleinberg, J.: Bursty and Hierarchical Structure in Streams, Proceedings of the Eighth ACM SIGKDD International Conference on Knowledge Discovery and Data Mining, 91-101, 2002.
Hidetsugu Nanba/Professor, Faculty of Science and Engineering, Chuo University
Area of Specialization: Natural Language Processing
Hidetsugu Nanba was born in Hiroshima Prefecture in 1972.
In 1996, he graduated from the Department of Electrical Engineering in the Faculty of Science and Technology, Tokyo University of Science.
In 2001, he completed the Doctoral Program in the School of Information Science, Japan Advanced Institute of Science and Technology. He holds a PhD in information science. He served as a Research Fellow for Young Scientists (Postdoctoral Researcher) at the Japan Society for the Promotion of Science; Assistant at the Precision and Intelligence Laboratory, Tokyo Institute of Technology; and Lecturer/Associate Professor in the Faculty of Information Sciences, Hiroshima City University. In 2019, he assumed his current position as Professor in Faculty of Science and Engineering, Chuo University.
He serves as a member of organizations including the Information Processing Society of Japan, the Association for Natural Language Processing, and the Japanese Society for Artificial Intelligence.
His current research themes include technology trend analysis for patents and academic papers, and traveler behavior analysis using travel blogs.